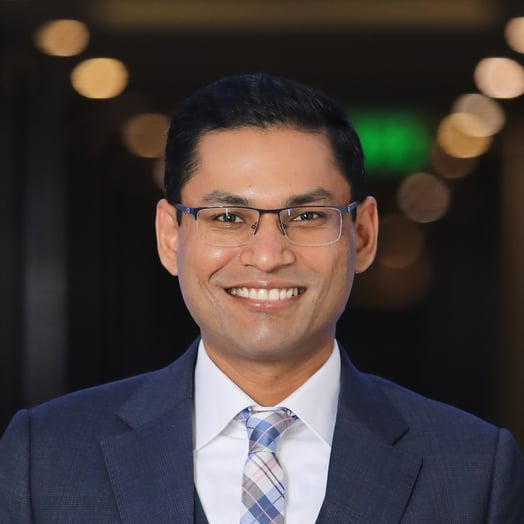
Naman Jain
Verified Expert in Engineering
数据工程架构师和首席开发人员
Naman is a highly experienced cloud and data solutions architect with more than six years of experience delivering data engineering services to multiple Fortune 100 clients. He has delivered on multiple Petabyte-scale data migrations and big data infrastructures via Azure Cloud, AWS Cloud, and Snowflake or DBT, 在许多情况下,在他们的用例中创建效率的阶梯顺序. 纳曼从根本上相信过度沟通, establishing trust, 并获得可交付成果的所有权.
Portfolio
Experience
Availability
Preferred Environment
Azure Cloud Services, Apache Spark, Scala, IntelliJ IDEA, Git, Linux, Snowflake, Data Build Tool (dbt), Snowpark, Data Migration
The most amazing...
...enterprise-grade, big data ELT platform I delivered in Azure Cloud was a single source of truth Data Lakehouse that enabled a wide diversity of use cases.
Work Experience
高级数据分析工程师
Enterprise Client
- Architected and delivered the clients' entire PROD logic lift (over 200 SQL workflows) and legacy data migration (over 10 Petabytes) from AWS Redshift to Snowflake.
- Automated the daily ingestion jobs via Data Building Tool (DBT) and created a self-updating data catalog via DBTCloud.
- 将所有SQL逻辑从Redshift SQL提升到DBT SQL. Used macros and Jinja, which allowed us to get visibility into very complex SQL logic and visualize it via the catalog.
- 缩短了80%的时间, cost, and real-time materialization of all our client-facing BI reports due to a cascading trigger in DBT, 而不是使用红移, 顺序表一次刷新.
- Linked all our Periscope charts and dashboards to a Git repo which was then indexed in an IDE. 这让我们能够制作并推送大量更新, replacing a manual logic updating process on Periscope and significantly increasing our efficiency.
- Trained the fresh data engineers to manage and extend this entire big data infrastructure.
- Compared performance, cost, and ease of maintenance of Snowpipe versus Fivetran versus Stitch.
- 在Snowpark中用Scala udf迁移并编写了一个新的非常复杂的业务逻辑. 通过简化Scala udf中的逻辑,帮助合并多个SQL表.
- 利用Snowpark建立了一个大数据平台, DBT, 和GitLab来实现最佳实践的标准化, CI/CD, 自我更新文档DAG, 减少金表新鲜度延迟, etc.
- Migrated over 10 Spark apps to Snowpark and achieved better net runtimes and reduced computing costs for all of them.
Cloud Solutions Architect
企业客户端(通过Toptal)
- 通过Azure数据工厂进行工作流的编排和自动化.
- 在Azure数据湖存储(ADLS) Gen2中优化和分区存储.
- Implemented complex, strongly-typed Scala Spark workloads in Azure Databricks along with dependency management and Git integration.
- Implemented real-time low cost and low latency streaming workflows which at their peak were processing more than 2MM raw JSON blobs per second. 通过ABS-AQS集成Azure Blob存储、Azure事件中心和Azure队列.
- Created a multi-layered ELT platform that consisted of raw/bronze (Azure Blob Storage), 当前和银(天蓝色三角洲湖), 和映射/金(天蓝色三角洲湖)层.
- Balanced the cost of computing by spinning up clusters on demand versus persisting them.
- Made big data available for efficient and real-time analysis throughout the client via Delta tables, 哪个提供了索引和优化的存储, ACID transaction guarantees, 以及表级和行级访问控制.
- Tied all together in end-to-end workflows that were either refreshed with just a few clicks or automated as jobs.
- Led a team of five consisting of four developers and one solutions architect to productionize big data workflows in Azure Cloud, enabling the client to sunset its legacy applications and experience far more reliable and scalable prod workflows.
- Enabled a wide diversity of use cases and future-proofed them by relying upon open source and open standards.
Lead Data Engineer
隐身模式AI初创公司(A轮2000万美元)
- 架构并实现了一个分布式机器学习平台.
- 通过Spark MLlib实现了20多个机器学习模型的产品化.
- Built products and tools to reduce time to market (TTM) for machine learning projects. 将初创公司从设计阶段到生产阶段的TTM减少了50%.
- Productionalized 8 Scala Spark applications to transform the ETL layer to feed into the machine learning models downstream.
- 使用Spark SQL进行ETL,使用Spark结构化流和Spark MLlib进行分析.
- 领导一个由三名数据科学家组成的六人团队, two back-end engineers, and one front-end engineer. Delivered a solution that had a back-end layer that talked to the front end via REST API and launched and managed Spark jobs on demand.
Senior Data Engineer
Dow Chemical (Fortune 62)
- Created five Scala Spark apps for ETL and wrote multiple Bash scripts for the automation of these jobs.
- Architected and built a Scala Spark app to validate Oracle source tables with their ingested counterparts in HDFS. The user can dynamically choose to conduct either a high-level or data-level validation.
- Developed the application so that its output would be the exact mismatched columns and rows between source and destination in case of a discrepancy.
- Reduced the engineer's manual debugging workload by over 99% by lowering it to just running the application and then reading the human-readable output file.
- 在预算范围内提前交付整个ETL和验证项目.
- 广泛使用Cloudera分布式Hadoop (CDH)用于HDFS和Hive.
Senior Data Engineer
波士顿科学公司(财富319)
- Designed and implemented a Scala Spark application to build Apache Solr indices from Hive tables. The app was designed for a rollback on any failure and reduced the downtime for downstream consumers from around 3 hours to around 10 seconds.
- Implemented a Spark Structured Streaming application to ingest data from Kafka streams and upsert them into Kudu tables in a Kerberized cluster.
- Set up multiple Shell scripts to automate Spark jobs, Apache Sqoop jobs, and Impala commands.
- 广泛使用Cloudera分布式Hadoop (CDH)和ElasticSearch.
Senior Data Engineer
通用磨坊(财富200强)
- 从各种来源获取社会营销数据, 包括Google Analytics API, Oracle Databases, 还有各种流媒体资源.
- Created a Scala Spark application to ingest >100Gb of data as a daily batch job, partition, 并以拼花的形式存储在HDFS中, 在查询层使用相应的Hive分区. 该应用程序取代了传统的Oracle解决方案,并将运行时间缩短了90%.
- 为ETL设置Spark SQL和Spark结构化流.
- 广泛使用Cloudera Distribution Hadoop (CDH).
Software Engineer
大都会人寿保险公司(财富44)
- 担任摩托车保险web应用程序的产品经理. 这款应用逐渐发展成为摩托车保险客户的主要着陆点.
- 生产前用于部署的构建主服务器. 部署了所有的构建,主要是构建的稳定性.
- 领导由30多名开发人员、测试人员和分析师组成的客户团队的Scrum开发.
- 在客户组织中架构和支持解决方案.
Experience
优化抵押贷款市场资金配置
http://github.com/Namanj/Mortgage-Market-Tri-Analysis我研究了舒巴姆住房金融公司的数据, a firm that has given out more than USD $150 million as mortgage loans over the past 5 years.
我的目标是利用数据科学来帮助公司优化其资本使用, 无论是在贷款分配过程中,还是在扩张过程中.
我决定把这个大目标分解成3个更具体的目标:
- Build a classifier that predicts the probability that a customer will default on their loan
-推荐新的办公地点,最大限度地提高增长潜力
-预测下一季度的业务量
Skills
Languages
Scala, SQL, Snowflake, Python 3, Bash
Frameworks
Spark, Apache Spark, Play Framework, Spark Structured Streaming, Hadoop, YARN
Libraries/APIs
Spark ML, MLlib, Google api
Tools
Git, IntelliJ IDEA, Spark SQL, Apache Impala, Apache Solr, Kudu, Apache Sqoop, Subversion (SVN), GitLab
Paradigms
ETL, ETL Implementation & Design, Functional Programming, Microservices Architecture, 面向对象编程(OOP), Agile Software Development, Agile, 模型-视图-控制器(MVC)
Platforms
Azure, Azure Event Hubs, Databricks, Linux, Apache Kafka, MacOS, Oracle Database
Storage
Data Lakes, Data Lake Design, Data Pipelines, Azure Cloud Services, Apache Hive, HDFS
Other
Azure Data Factory, Azure Data Lake, Data Engineering, Data Warehousing, Delta Lake, Data Migration, Azure Data Lake Analytics, ETL Development, Big Data, Data Architecture, Big Data Architecture, ELT, Azure Databricks, Data Warehouse Design, Data Build Tool (dbt), Machine Learning, Data Structures, Snowpark
Education
计算机科学与工程学士学位
美国俄亥俄州哥伦布市俄亥俄州立大学
Certifications
Spark and Hadoop Developer
Cloudera
Data Science Bootcamp
镀锌|美国加州旧金山
How to Work with Toptal
Toptal matches you directly with global industry experts from our network in hours—not weeks or months.
Share your needs
Choose your talent
开始你的无风险人才试验
对顶尖人才的需求很大.
Start hiring